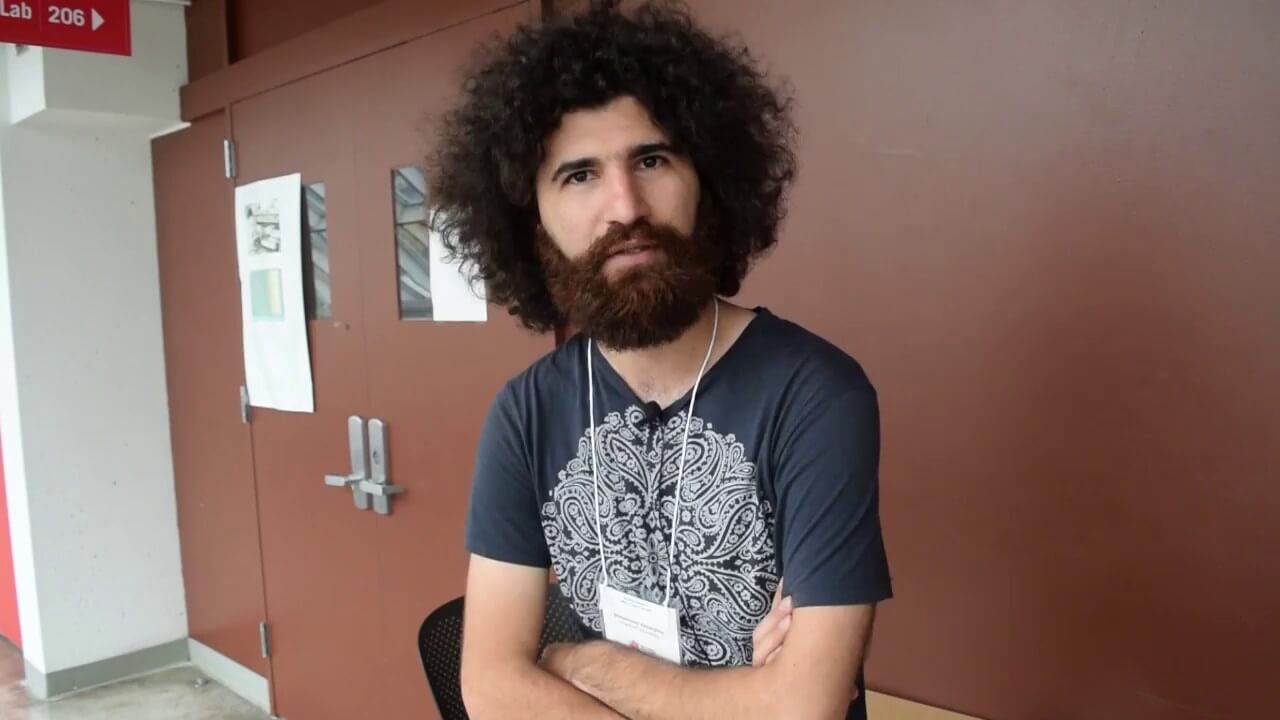
Title: Information aggregation in overlapping generations and the emergence of experts
Abstract: We study a model of social learning with “overlapping generations”, where agents meet others and share data about an underlying state over time. We examine under what conditions the society will produce individuals with precise knowledge about the state of the world. There are two information sharing regimes in our model: Under the full information sharing technology, individuals exchange the information about their point estimates of an underlying state, as well as their sources (or the precision of their signals) and update their beliefs by taking a weighted average. Under the limited information sharing technology, agents only observe the information about the point estimates of those they meet, and update their beliefs by taking a weighted average, where weights can depend on the sequence of meetings, as well as the labels. Our main result shows that, unlike most social learning settings, using such linear learning rules do not guide the society (or even a fraction of its members) to learn the truth, and having access to, and exploiting knowledge of the precision of a source signal are essential for efficient social learning (joint with Amin Saberi & Ali Shameli).