Abstract: Many statistical and computational tasks boil down to comparing probability measures expressed as density functions, clouds of data points, or generative models. In this setting, we often are unable to match individual data points but rather need to deduce relationships between entire weighted and unweighted point sets. In this talk, I will summarize our team’s recent efforts to apply geometric techniques to problems in this space, using tools from optimal transport and spectral geometry. Motivated by applications in dataset comparison, time series analysis, and robust learning, our work reveals how to apply geometric reasoning to data expressed as probability measures without sacrificing computational efficiency.
Geometric Models for Sets of Probability Measures
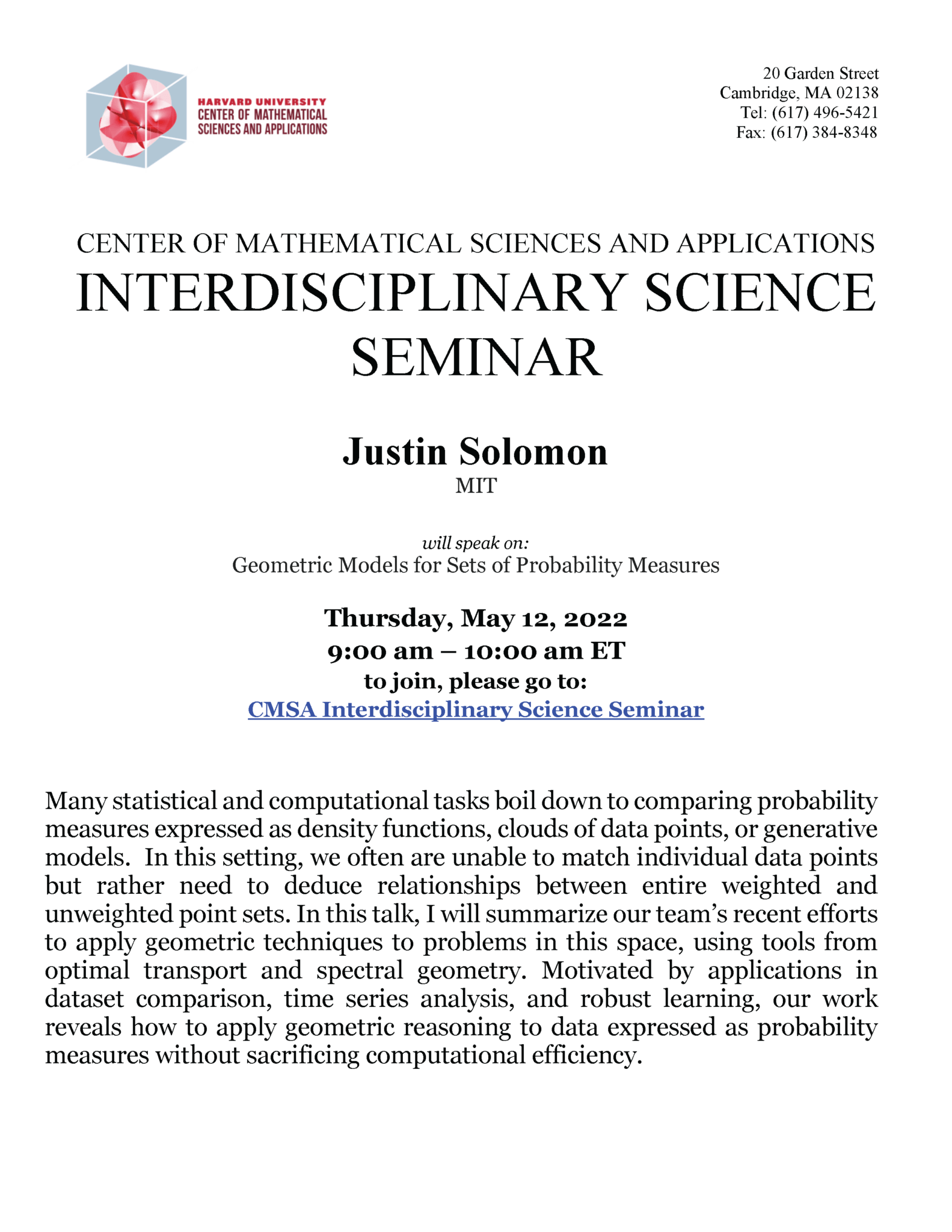
05/12/2022 3:38 pm - 5:38 pm