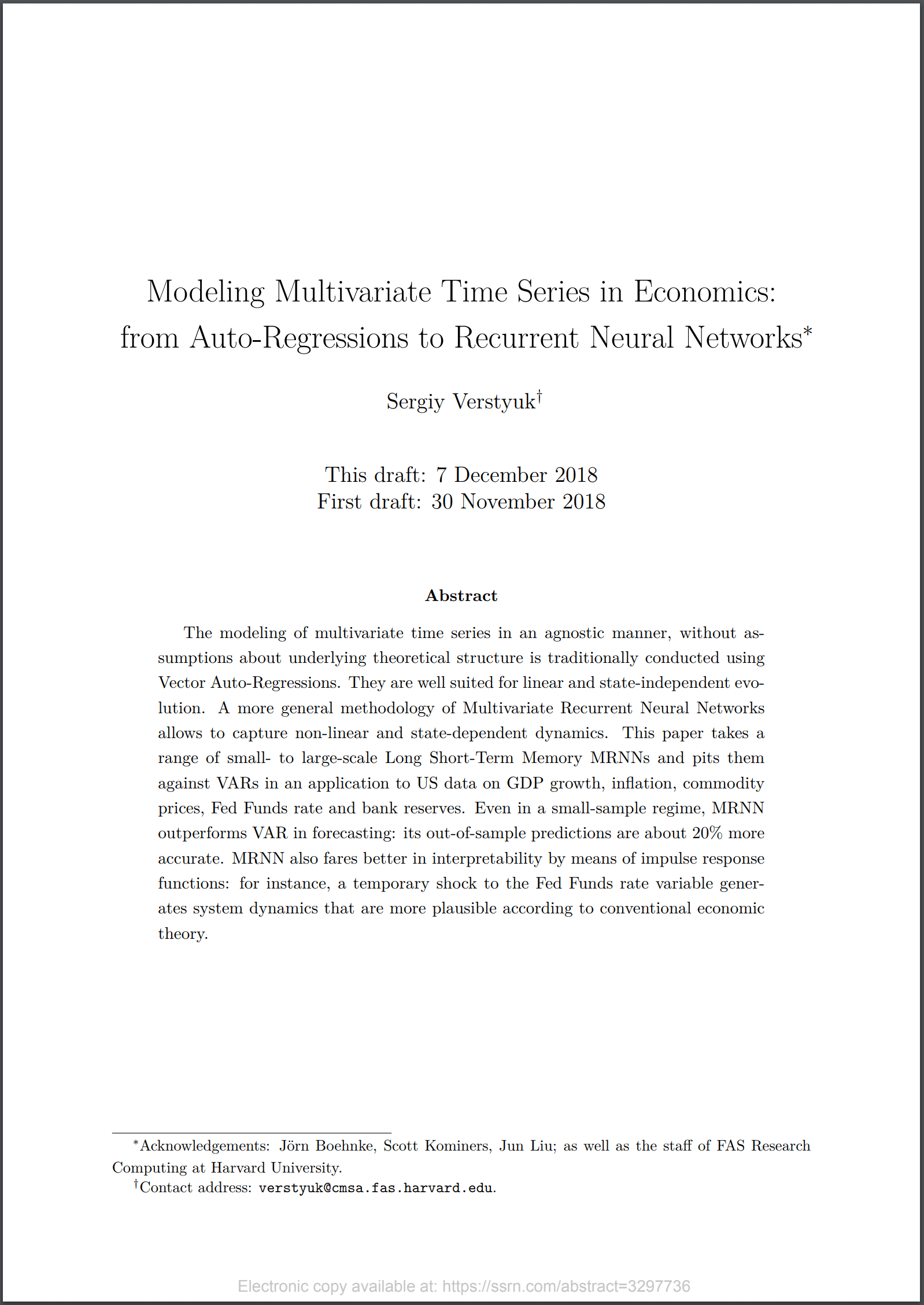
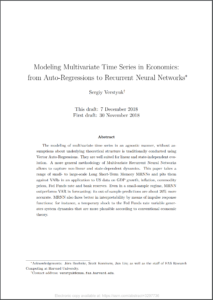
A new paper by Sergiy Verstyuk:
Abstract: The modeling of multivariate time series in an agnostic manner, without assumptions about underlying theoretical structure is traditionally conducted using Vector Auto-Regressions. They are well suited for linear and state-independent evolution. A more general methodology of Multivariate Recurrent Neural Networks allows to capture non-linear and state-dependent dynamics. This paper takes a range of small- to large-scale Long Short-Term Memory MRNNs and pits them against VARs in an application to US data on GDP growth, inflation, commodity prices, Fed Funds rate and bank reserves. Even in a small-sample regime, MRNN outperforms VAR in forecasting: its out-of-sample predictions are about 20% more accurate. MRNN also fares better in interpretability by means of impulse response functions: for instance, a temporary shock to the Fed Funds rate variable generates system dynamics that are more plausible according to conventional