
- This event has passed.
Big Data Conference 2022
August 26, 2022 @ 9:00 am - 1:00 pm
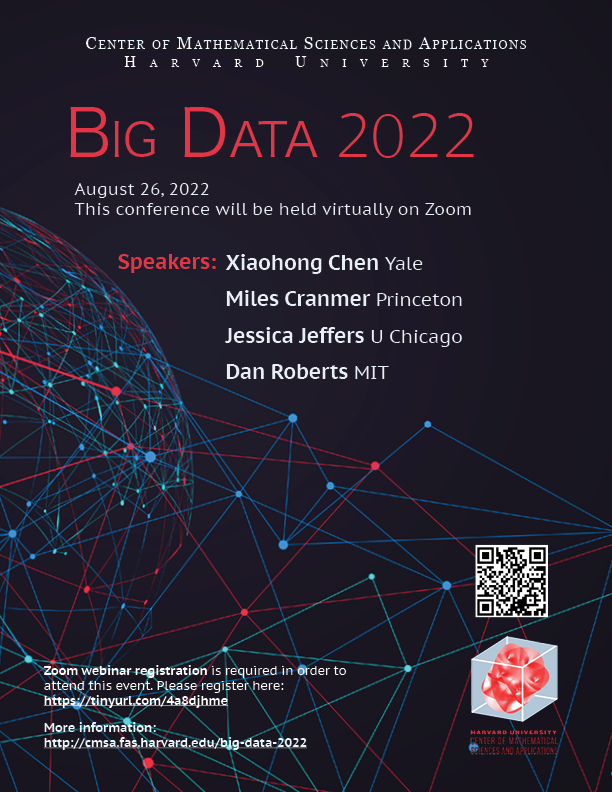
On August 26, 2022 the CMSA hosted our eighth annual Conference on Big Data. The Big Data Conference features speakers from the Harvard community as well as scholars from across the globe, with talks focusing on computer science, statistics, math and physics, and economics.
The 2022 Big Data Conference took place virtually on Zoom.
Organizers:
- Scott Duke Kominers, MBA Class of 1960 Associate Professor, Harvard Business
- Horng-Tzer Yau, Professor of Mathematics, Harvard University
- Sergiy Verstyuk, CMSA, Harvard University
Speakers:
- Xiaohong Chen, Yale
- Miles Cranmer, Princeton
- Jessica Jeffers, University of Chicago
- Dan Roberts, MIT
Schedule
9:00 am | Conference Organizers | Introduction and Welcome |
9:10 am – 9:55 am | Xiaohong Chen | Title: On ANN optimal estimation and inference for policy functionals of nonparametric conditional moment restrictions
Abstract: Many causal/policy parameters of interest are expectation functionals of unknown infinite-dimensional structural functions identified via conditional moment restrictions. Artificial Neural Networks (ANNs) can be viewed as nonlinear sieves that can approximate complex functions of high dimensional covariates more effectively than linear sieves. In this talk we present ANN optimal estimation and inference on policy functionals, such as average elasticities or value functions, of unknown structural functions of endogenous covariates. We provide ANN efficient estimation and optimal t based confidence interval for regular policy functionals such as average derivatives in nonparametric instrumental variables regressions. We also present ANN quasi likelihood ratio based inference for possibly irregular policy functionals of general nonparametric conditional moment restrictions (such as quantile instrumental variables models or Bellman equations) for time series data. We conduct intensive Monte Carlo studies to investigate computational issues with ANN based optimal estimation and inference in economic structural models with endogeneity. For economic data sets that do not have very high signal to noise ratios, there are current gaps between theoretical advantage of ANN approximation theory vs inferential performance in finite samples. The talk is based on two co-authored papers: (2) Neural network Inference on Nonparametric conditional moment restrictions with weakly dependent data |
10:00 am – 10:45 am | Jessica Jeffers | Title: Labor Reactions to Credit Deterioration: Evidence from LinkedIn Activity
Abstract: We analyze worker reactions to their firms’ credit deterioration. Using weekly networking activity on LinkedIn, we show workers initiate more connections immediately following a negative credit event, even at firms far from bankruptcy. Our results suggest that workers are driven by concerns about both unemployment and future prospects at their firm. Heightened networking activity is associated with contemporaneous and future departures, especially at financially healthy firms. Other negative events like missed earnings and equity downgrades do not trigger similar reactions. Overall, our results indicate that the build-up of connections triggered by credit deterioration represents a source of fragility for firms. |
10:50 am – 11:35 am | Miles Cranmer | Title: Interpretable Machine Learning for Physics
Abstract: Would Kepler have discovered his laws if machine learning had been around in 1609? Or would he have been satisfied with the accuracy of some black box regression model, leaving Newton without the inspiration to discover the law of gravitation? In this talk I will explore the compatibility of industry-oriented machine learning algorithms with discovery in the natural sciences. I will describe recent approaches developed with collaborators for addressing this, based on a strategy of “translating” neural networks into symbolic models via evolutionary algorithms. I will discuss the inner workings of the open-source symbolic regression library PySR (github.com/MilesCranmer/PySR), which forms a central part of this interpretable learning toolkit. Finally, I will present examples of how these methods have been used in the past two years in scientific discovery, and outline some current efforts. |
11:40 am – 12:25 pm | Dan Roberts | Title: A Statistical Model of Neural Scaling Laws
Abstract: Large language models of a huge number of parameters and trained on near internet-sized number of tokens have been empirically shown to obey “neural scaling laws” for which their performance behaves predictably as a power law in either parameters or dataset size until bottlenecked by the other resource. To understand this better, we first identify the necessary properties allowing such scaling laws to arise and then propose a statistical model — a joint generative data model and random feature model — that captures this neural scaling phenomenology. By solving this model using tools from random matrix theory, we gain insight into (i) the statistical structure of datasets and tasks that lead to scaling laws (ii) how nonlinear feature maps, i.e the role played by the deep neural network, enable scaling laws when trained on these datasets, and (iii) how such scaling laws can break down, and what their behavior is when they do. A key feature is the manner in which the power laws that occur in the statistics of natural datasets are translated into power law scalings of the test loss, and how the finite extent of such power laws leads to both bottlenecks and breakdowns. View/Download Lecture Slides (pdf)
|
12:30 pm | Conference Organizers | Closing Remarks |
Information about last year’s conference can be found here.